Novel Machine Learning Techniques Measure Ocean Oxygen Loss More Accurately
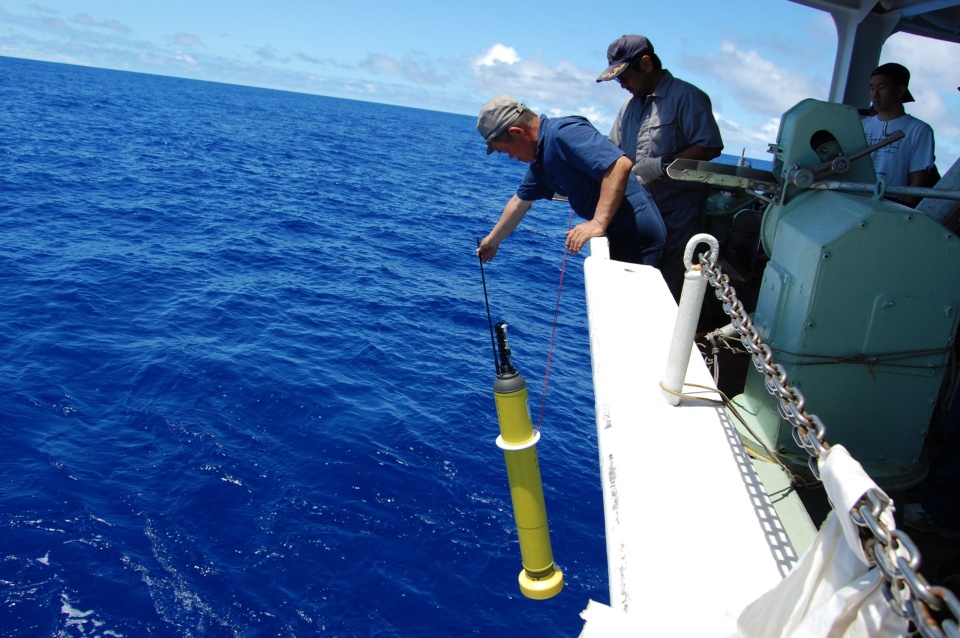
Using data from Argo floats (pictured) and historic ship measurements, Georgia Tech researchers developed new machine learning techniques to better understand global ocean oxygen loss. (Credit: Argo Program, UCSD)
Oxygen is essential for living organisms, particularly multicellular life, to metabolize organic matter and energize all life activities. About half of the oxygen we breathe comes from terrestrial plant life, such as forests and grasslands, while the other half is produced through photosynthesis by marine algae in the ocean's surface waters.
Oxygen concentrations are declining in many parts of the world’s oceans. Experts believe this drop is linked to the ocean’s surface warming and its impacts on the physics and chemistry of seawater, though the problem is not fully understood. Temperature plays a crucial role in determining how oxygen dissolves in seawater; as water warms, it loses its ability to hold gas.
“Calculating the amount of oxygen lost from the oceans is challenging due to limited historical measurements and inconsistent timing,” said Taka Ito, oceanographer and professor in the School of Earth and Atmospheric Sciences at Georgia Tech. “To understand global oxygen levels and their changes, we need to fill in many data gaps.”
A group of student researchers sought to address this issue. Led by Ito, the team developed a new machine learning-based approach to more accurately understand and represent the decline in global ocean oxygen levels. Using datasets, the team further generated a monthly map of oxygen content visualizing the ocean’s oxygen decline over several decades. Their research was published in the Journal of Geophysical Research: Machine Learning and Computation.
“Marine scientists need to understand the distribution of oxygen in the ocean, how much it's changing, where the changes are occurring, and why,” said Ahron Cervania, a Ph.D. student in Ito’s lab. “Statistical methods have long been used for these estimates, but machine learning techniques can improve the accuracy and resolution of our oxygen assessments.”
The project began three years ago with support from the National Science Foundation, and the team initially focused solely on Atlantic Ocean data to test the new method. They used a computational model to generate hypothetical observations, which allowed them to assess how well they could reconstruct missing oxygen level information using only a fraction of the data combined with machine learning. After developing this method, the team expanded to global ocean observations, involving undergraduate students and delegating tasks across different ocean basins.
Under Ito’s guidance, Cervania and other student researchers developed algorithms to analyze the relationships between oxygen content and variables like temperature, salinity, and pressure. They used a dataset of historical, ship-based oxygen observations since the 1960s and recent data from Argo floats — autonomous drifting devices that collect and measure temperature and salinity. Although oxygen data existed before the 1960s, earlier records have accuracy issues, so the team focused on data from the 1960s onward. They then created a global monthly map of ocean oxygen content from 1965 to the present.
“Using a machine learning approach, we were able to assess the rate of oxygen loss more precisely across different periods and locations,” Cervania said. “Our findings indicate that incorporating float data significantly enhances the estimate of oxygen loss while also reducing uncertainty.”
The team found that the world’s oceans have lost oxygen at a rate of about 0.7% per decade from 1970 to 2010. This estimate suggests a relatively rapid ocean response to recent climate change, with potential long-term impacts on marine ecosystems’ health and sustainability. Their estimate also falls within the range of decline suggested by other studies, indicating the accuracy and efficacy of their approach.
“We calculated trends in global oxygen levels and the ocean’s inventory, essentially looking at the rate of change over the last five decades,” Cervania said. “It’s encouraging to see that our rate aligns with previous estimates from other methods, which gives us confidence. We are building a robust estimate from both our study and other studies.”
According to Ito, the team’s new approach addresses an ongoing challenge in the oceanographic community: how to effectively combine different data sources with varying accuracies and uncertainties to better understand ocean changes.
“The integration of advanced technologies like machine learning will be essential in filling data gaps and providing a clearer picture of how our oceans are responding to climate change.”
Citation: Ito, T., Cervania, A., Cross, K., Ainchwar, S., & Delawalla, S. (2024). Mapping dissolved oxygen concentrations by combining shipboard and Argo observations using machine learning algorithms. Journal of Geophysical Research: Machine Learning and Computation, 1, e2024JH000272.
DOI: https://doi.org/10.1029/2024JH000272
Funding: National Science Foundation
Additional Media
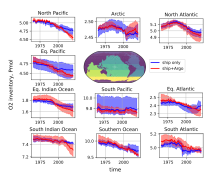
Basin-scale oxygen inventory trend with global ocean divided to 10 basins. Blue lines and shading show ensemble mean and ensemble range for ship-only reconstructions, and red lines and shading are for ship and Argo float reconstructions. The research team divided up the task by working on different basins. (Credit: Georgia Institute of Technology)
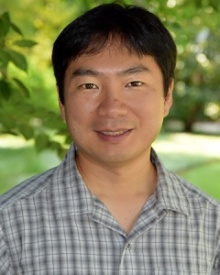
Taka Ito, oceanographer and professor in the School of Earth and Atmospheric Sciences.
Ahron Cervania, a Ph.D. student in Earth and Atmospheric Sciences.